By Gayan Gunewardana, Bsc/MBA/CIMA/CGMA/AIB/FRM (Banking consultant & independent researcher – Middle East)
The global “war” on COVID-19 pandemic and related economic impact most certainly could lead to a global recession. This will push further 500m people into extreme poverty as per estimates. Despite, the number of initiatives by governments in terms of new regulations and stimulus packages, still may actions to be done yet to avoid falling these economies into “life-support”. Overall, The WTO said global trade would fall this year by 13%-32%. Thus COVID-19 or COVID-Economy would bring the largest systemic – risk unification the world has ever comprehended. In finance, the ‘systemic risk’ is explained as the downside (risk) of collapsing the entire financial system or a major industry. Theoretically, during excessive risk scenarios, the established correlations tend to change, increase the volatilities or the correlation volatility can be changed as well. Risk Rating agencies expect to have the capacity to identify these new norms. The Systemic Risk Council (SRC) has clearly communicated to G-20, the key contributors of systemic -risk escalations due to pandemic situation. As per there statement, “COVID-19 strikes at a time when too many corporations are indebted, and after a period during which persistently favorable market conditions caused traders to take aggressive positions, exposing them and the system to spikes in volatility, let alone a collapse in assets value”.

The role of external- credit rating agencies (CRA) in the modern financial system has been well recognized. The rating models are mainly engaged in ascertaining the creditworthiness of a counterparty or an issue of financial instruments like bonds and other debt instruments. The regulatory modifications taken place with the introduction of the Basel capital accord, have enhanced the prominence of these external rating systems rapidly. Thus Risk -ratings directly correlates with the required capital adequacy levels or decides the Expected Credit Loss (ECL). Currently, the demands of IFRS-9 contemplates the deteriorating in risk rating as a critical indicator against creditworthiness or on ECL. In the eyes of the regulators, the risk rating settles the informative asymmetry between the lender and the potential borrower on the credit standings. Simply, the rating models compress a large number of judgmental and qualitative information, in order to derive the ultimate grading. Consequently, a fund-seeker with a strong rating enjoys better pricing, comparatively lenient credit terms/conditions and low securities’ requirements from the lender. The global rating industry is presently highly concentrated, thus the prominent players relish a strong oligopolistic status, predominantly due to their reputation and vintage. The internationally renowned rating agencies like Standard and Poor (S&P), Fitch and Moodys provide diverse risk classifications and different architectures, though ultimate readings are mostly in the same value array. Obligor with top-AAA rated by S&P can most likely obtain AAA from Fitch or Aaa rating from Moody’s, which represent similar risk standing within top investment notch. These solid risk ratings along with strong corporate governance structure, solid business projections help the entrepreneurs to access robust global capital markets with low-cost funding irrespective of their domicile.
M/s Fitch explains AAA as “the best quality investment-grade companies, reliable and stable”. Thus the credit rating essentially represents the probability of default (PD) or the level of credit risk. These projections within the selected time horizon is an important component in capital planning and other budgetary requirements of any modern lender. Hence, PDs are expected to have a solid accuracy level to make these estimations more realistic. Expressly, during the Asian financial crisis between1997-1998 and following the 2008-2009 global financial crisis, the risk ratings and role of CRA were criticized largely. Simply, most of such grades were lagging behind and unable to identify the possible defaults associated with these leading market catastrophes. The risk rating failure in identifying the systemic risk has resulted in dwindling the dependability on enterprise risk management (ERM) systems especially during these crises. As one of the principal objectives of ERM is to recognize the customer defaults proactively with the sensible comfort level. Hence, the absolute failure in core risk management tools has become the center of discussion among the financial fraternity. On the other hand, these models due to subsequent modifications have started to overreact negativity after the post-crisis. Both causes have contributed largely in extending these weak economic cycles further. This counter- productivity has almost damaged the reliability of rating systems by the specialized lenders for credit decision-making. Hence, consequently fueled the chronological thinking of going back to personal judgments or “gut- feeling” based assessments as an alternative. This on the more specific way has necessitated the agencies to reconsider the possibilities of recalibrating with more market base elements to the models. The urgency is also to re-visit the analytical methods and use more advanced statistical measurements for projections. On the other hand, appeals came from many environments to unveil the baseline assumptions and back-end calculations on their respective risk methodologies. However, still, the transparency levels are decided exclusively by these agencies internally in view of their large investment towards developing these intellectual properties. As per the assumption, more transparency and disclosures might draw more competitors towards the industry and also could lead to more widespread debates on the flaws of the models.

The failure of risk rating models in identifying the clustering defaults has been well documented and the same has exposed all top rating models noticeably. Accordingly, the incapability to predict the largescale defaults within a short span of time is the prevalent challenge. COVID-19 has posted similar sanity- tests currently. The core failure directly represents the weaknesses in capturing systemic risk again. However, a strong mechanism has been developed to recheck the model’s accuracy lately through advanced backtesting techniques. Backtesting is the methodology engaged to validate the accuracy levels of these models or to have clarity on model would have done ex-post. Most system specialists use three components on a combined basis to perform backtesting. Explicitly, the distance to defaults (against the original probability of default), pre-identification of declining behavior/ trend and thirdly, the (arrived) final rating prior to actual default or bankruptcy. These techniques are universally employed for the risk-model assessments as these are good testing components (of defaults).
A random sample of 64 corporates’ defaults reported since 2016 covering some advanced and emerging markets were selected for the below backtesting purpose. The statistics are interesting and are summarized to below bar chart.
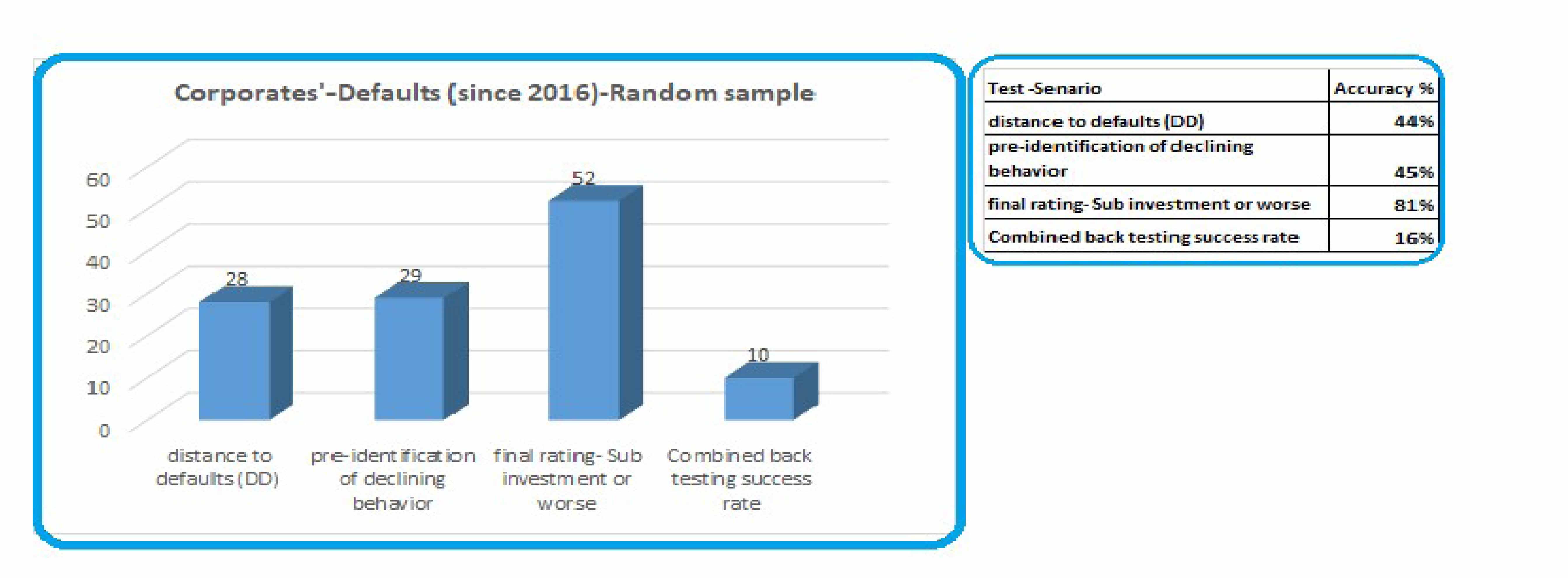
As per the above graph, fittingly the most of the cases prior to actual defaults were classified as subinvestment or worst ratings (81%). The other two test scenarios have not performed as expected and both fallen below 50% accuracy level. The overall accuracy level combining all three critical parameters remained at moderate 16% which is the reflection of major drawback. The universal- benchmark for model acceptability is above 70% for backtesting conditions. Also to note that in a few observed cases, the respective risk agencies have withdrawn the given rating after the bankruptcies were actually filed. They cannot be considered as systemic downgrading, than a mere effort to avoid reputation risk.
The most infamous withdrawals were reported in 2008 by Moody’s on Lehman Brothers, prior to chapter 11 bankruptcy filing. The said bankruptcy was a direct result of unprecedented losses due to the continuing subprime mortgage crisis, which failed to capture throughout the cycle. Lehman's losses resulted from having held onto large positions in subprime and other lower-rated mortgage tranches when securitizing the underlying mortgages. As per Lehman’s sources, they also heavily relied on the risk rating of underlined assets for the building up of portfolios from undervalued assets. The time has proved that the risk rating on these assets too was profoundly wrong. This inflated credit- ratings had eventually led to massive mispricing of risk and hence overpricing of the captioned assets. That state was shockingly introduced as “rating- inflations” by many financial critiques. The rating parameters were here incompetent either in understanding the correlations with the macro elements and/or the change in the nature of the relationships (correlation- volatility). However, as an eminent exception, historically the rating systems have successfully captured the probability of oil-related defaults with a high confidant level. The oil trading sector in the West African region is a worthy example as explained earlier. The accuracy rate therein was in the high 80s, despite the oil companies’ failures were largely linked to many macro variables. Some of these variables include the political-price-cost-geological –supply and demand risks, etc. However, in the broad sense, most of the oil and gas players are exposed to an identical systemic-risk which as per experts is easily predictable. Small and medium-sized enterprise credit analysis has a different dimension. Most of the SME lenders popularly use the rating- based -scoring –methods (RBSM), not only for the capital adequacy calculations/ planning purposes but also for the basis of important credit decisions. The applications are filtered through scoring methods are considered as “good credit” and rest would be reviewed or declined. As per historical evidence and in line with the writer’s chosen sample, these scoring models too have performed poorly during the times of economic downturns. In fact, this has become countercyclical and contributes to the major small-lending-banking sector failure. The basic assumptions of these methods are elementary, the future would be like the past without major change, is fundamentally incorrect. Accordingly, the same set of key financial and non-financial parameters being used for subjective analysis, are essentially weak and remain constant both during the economic boom and dip. Mostly, these models on small-ticket sizes would not keep any leeway for expert opinions or manipulation based on contemporary market changes. Not to mention that the SME rating failure has also largely been contributed by fragmental financial data, low controls, corporate governance and personal bias of the promoter, etc.

The drifting of risk-models are largely due to the limited use of more dominant and directly connected - macro elements in most cases. The commonly used hard information mainly consists of financial data and other measurable quantitative elements like years of existence, debtors concentration, adherence to set covenants and conditions, etc. The hard information is generally obligor specific and would not explain the data pertaining to the broad geopolitics outlook. Some in-depth studies even have pointed out that the weaknesses within the current hard-information space as well. As an illustration, more accurate and highly impacted investor specific ratios have rarely been integrated into these models. On the other hand, the soft and non-quantifiable rudiments like management- quality, perception, specialization, strength/ weaknesses in value -chain, long-term corporate planning and ability to generate funding, etc, have either not been represented properly or dispense exclusively for the personal judgment of the credit officer without proper scientific evaluation. The most demanding proposition from these findings is that, to use the projected macro scenarios to stress the financial performance/cash- flows and business projections, in order to assess the sustainability of the business model in the future. As an alternative, the high-level ratios like margin at risk, earnings at risk and cash-flow at risk can be compared with the external -debt (financing) and future maturities to gage the obligor’s ability to survive more diligently.
The US banking sector is the first to introduce regulatory- guidelines for model-risk verification and validation (in the US). Also, the use of IFRS: 9, standard on impairment, mandates the banks to use a comprehensive rating methodology even as a part of the Internal Risk-Based (IRB) regime. Lately, many banks have taken these monitory impacts of (wrong) selection and model-risk failures as a part of their model risk limits within the overall risk appetite framework, which was not an exercise before. Overall, many aspects of Risk modeling architecture is yet to be upgraded. The model scope is to be extensive. And same should capture all possible macro and macro elements that are relevant and have direct or significant indirect influences over the creditworthiness of the obligor. The model life cycle efficiency level or the time taken for the contemporary adjustments on receipt of new information is to be shorter. The risk-governance aspect is to be strengthened with the support of the board and consistent commitment from the top- management. The regulators can link their supervision mechanism and validation process towards achieving these border intents. As a starter, they can showcase the better examples of more sophisticated banks’ core understanding and experiences with weaker opponents, who are lagging behind the process. Vibrant awareness is to be promoted towards considering the trusted and tested risk-models as an integral part of the enterprise-strategic assets in risk management. Accordingly, the model-risk management should not only be considered as an obligatory requirement but also as a key tool to add value to the credit evaluation process on a large scale. These models can be leveraged ultimately to achieve extensive corporate objectives and to secure valuable capital to the banks' owners. The wider need for data scientists in identifying these historical trends and in designing the predictive models, proper backtesting and hypnotical stressed tests can be paramount to achieve these objectives in the long run. Accordingly, it is interesting to know how these CRAs manage to overcome the reality check associated with COVID-19–Economy and present meaningful rating and risk architecture to stakeholders in the commercial world.